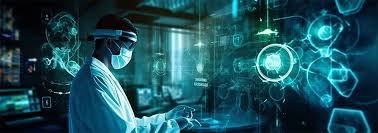
Table of Contents
- Introduction to NLP and AI in Medicine
- The Role of NLP AI in Drug Discovery
- Benefits of NLP AI in Drug Discovery
- Challenges and Limitations
- Future Prospects
- How to Get Started with NLP AI
- Conclusion
Introduction to NLP and AI in Medicine
Advancements in NLP and AI have led to new possibilities in various sectors, particularly drug development. AI drug discovery leverages these technologies to analyze large datasets, uncovering insights that accelerate the medical research process. NLP, a branch of AI, focuses on the interaction between computers and human language, making it an essential tool for sifting through vast amounts of biomedical literature. This interplay between NLP and AI enables researchers to quickly identify and act on relevant information, ultimately transforming the pace at which new medicines are discovered and developed.
The Role of NLP AI in Drug Discovery
The traditional process of discovering drugs is known for being both time-consuming and costly, typically requiring years and billions of dollars to introduce a new medication to the market successfully. The extended duration is mainly because of the thorough research and multiple stages of testing needed to guarantee a drug’s effectiveness and safety. However, the integration of NLP AI can significantly streamline this process. By automating the analysis of scientific literature, patents, clinical trial records, and other textual data, NLP AI aids researchers in identifying potential drug candidates faster and more efficiently. This accelerated analysis saves time and allows for a more comprehensive examination of available data, increasing the likelihood of identifying promising drug candidates earlier in the process.
- Automated Data Analysis: NLP AI algorithms can quickly scan and interpret vast amounts of data, identifying critical information that can be used to discover new drug compounds. For instance, these algorithms can analyze existing research papers to find correlations between diseases and potential treatments that human researchers may have overlooked. This capability is precious when researchers search for initial leads in the early drug discovery stages. By rapidly processing large datasets, NLP AI tools provide a rich source of information that can inform hypothesis generation and experimental design.
- Predictive Modeling: Another critical application is predictive modeling. NLP AI platforms can replicate the interactions between drug compounds and biological targets, enabling researchers to anticipate the effectiveness and safety of new treatments before participating in clinical trials. This approach saves time and reduces the financial risk associated with drug development. By generating accurate predictions about a compound’s interactions and potential side effects, predictive modeling helps prioritize the most promising candidates for further testing, thereby streamlining the drug development pipeline.
Benefits of NLP AI in Drug Discovery
- Efficiency: Automated data analysis reduces the time and human resources required for initial drug candidate identification. By automating repetitive tasks, researchers can focus on more complex aspects of drug discovery, enhancing overall productivity.
- Cost-Effectiveness: By accelerating the early stages of drug discovery, companies can save significant money. Reduced timeframes and fewer required resources translate into lower overall costs, making the drug development process more economically viable.
- Enhanced Accuracy: NLP AI technology can identify patterns and relationships that human researchers may overlook, resulting in more precise forecasts. These accurate observations allow for better decision-making, improving the chances of successful drug development.
Challenges and Limitations
Although NLP AI has many advantages, it also has its restrictions. The AI’s performance is greatly influenced by the amount and quality of data used for training. Additionally, the intricate characteristics of biological systems require that AI predictions be confirmed through experimental and clinical trials. Ethical concerns about data privacy and the risk of bias in AI algorithms are also worth considering. These difficulties emphasize the importance of continuous research and improvement to guarantee the reliability and ethics of NLP AI tools.
- Data Quality: One of the most significant challenges is the data quality being used. AI algorithms are only as good as the data they are trained on. Consistent or incomplete datasets can lead to accurate predictions, derailing drug discovery efforts. Ensuring high-quality, comprehensive datasets is crucial for the success of NLP AI applications. Data curation and preprocessing steps are essential to maximize the utility of available information and enhance the accuracy of AI-driven insights.
- Ethical and Regulatory Concerns: Another obstacle arises from ethical and regulatory issues. Stringent regulatory standards must be followed to ensure patient safety when using AI in drug discovery. Moreover, it is essential to tackle ethical concerns regarding data privacy and the risk of algorithmic bias. As AI technologies are increasingly incorporated into drug discovery processes, upholding transparency, accountability, and ethical integrity will be crucial. Regulatory agencies and those involved in the industry must work together to create strong rules that safeguard the patients’ interests while still allowing for innovation.
Future Prospects
The future of NLP AI in drug discovery is promising. The scope for NLP AI applications is broadened with continuous advancements in AI technologies and increasing volumes of biomedical data. Innovations such as deep learning and generative models promise to enhance AI’s capabilities in this field further. These cutting-edge technologies can offer more sophisticated analytical tools, enabling more profound insights and accurate predictions. As the AI landscape evolves, its integration into drug discovery will likely yield even more transformative impacts, driving medical advancements at an unprecedented pace.
How to Get Started with NLP AI
For institutions and companies interested in implementing NLP AI in their drug discovery processes, start by investing in robust data infrastructure and employing skilled data scientists. Building a solid technical foundation is essential for leveraging the full potential of NLP AI tools. Collaborating with academic institutions and technology companies is advantageous for remaining on top of AI advancements. These partnerships can facilitate knowledge sharing, access to cutting-edge technology, and collaborative research efforts. Additionally, keeping up with industry standards and regulations is essential to ensure AI technologies’ ethical and practical use. Following established protocols and meeting regulatory standards can assist organizations in successfully implementing AI while upholding ethical principles.
Conclusion
NLP AI is genuinely revolutionizing the field of drug discovery. The potential benefits are vast, from reducing time and cost to enhancing accuracy and efficiency. As technology keeps advancing, its use in medicine will also progress, providing promising possibilities for the future of healthcare. By adopting these advancements, the pharmaceutical industry can enhance patient results and introduce cutting-edge treatments to the market faster, ultimately reshaping the future of medicine.